
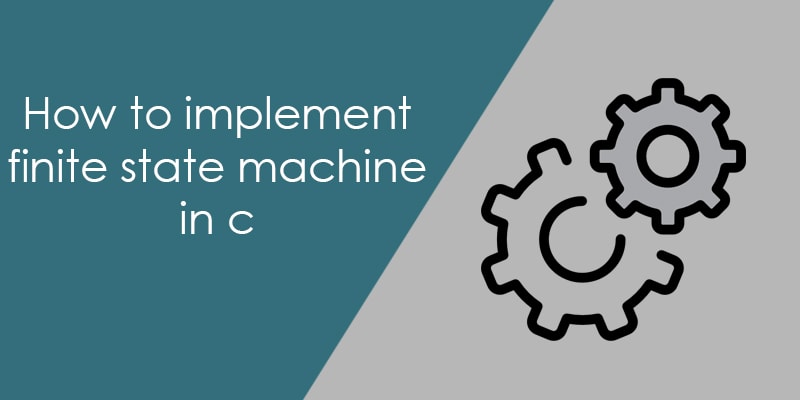
In: Proceedings of IEEE international conference on neural networks. Kennedy J, Eberhart R (1995) Particle swarm optimization. Politecnico di Milano, Italy, Technical Report, Report No. MIT Press, Cambridgeĭorigo M, Maniezzo V, Colorni A (1991) Positive feedback as a search strategy. Koza JR (1992) Genetic programming on the programming of computers by means of natural selection. Sumathi S, Ashok Kumar L, Surekha P (2015) Computational intelligence paradigms for optimization problems using Matlab/Simulink.
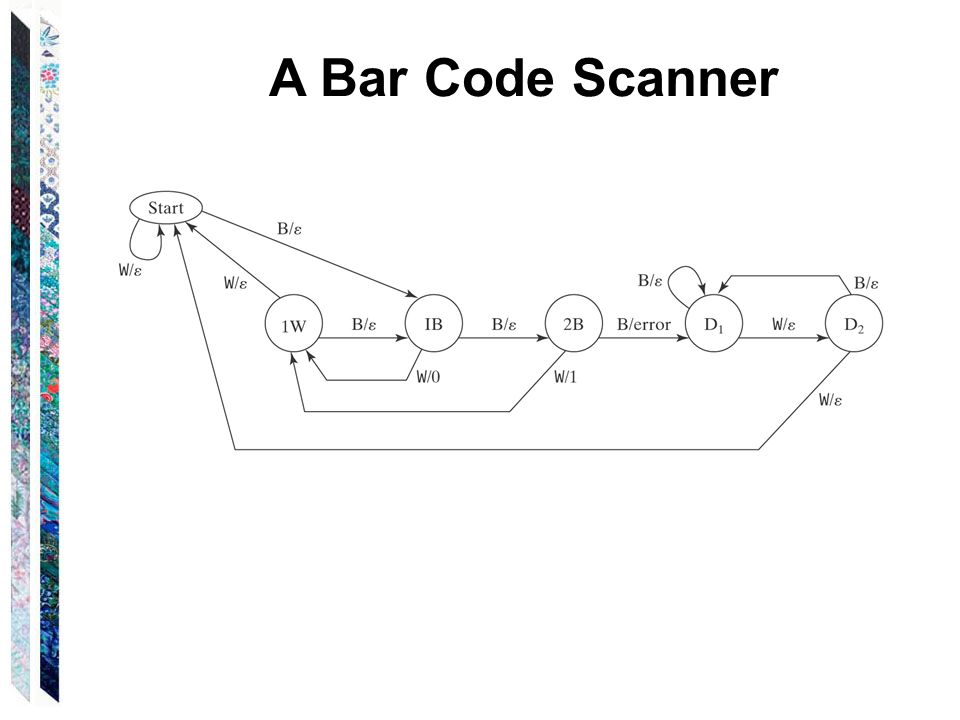
Konar A (2005) Computational intelligence: principles, techniques and applications. Liu T, Liu AX, Shi J, Sun Y, Guo Li (2014) Towards fast and optimal grouping of regular expressions via DFA size estimation. In: Proceedings of the 7th IEEE/ACM international conference on hardware/software codesign and system synthesis. Rohrer J, Atasu J, Van Lunteren J, Hagleitner C (2009) Memory-efficient distribution of regular expressions for fast deep packet inspection. University of California at Berkeley, Technical Report No.UCB/EECS-2006-76. Yu F, Chen Z, Diao Y, Lakshman TV, Katz RH (2006) Fast and memory-efficient regular expression matching for deep packet inspection.
#Finite state automata put back series
Addison-Wesley Series in Computer Science. Hopcroft JE, Motwani R, Ullman JD (2001) Introduction to automata theory, languages, and computation, 2nd edn. Nitin T, Singh SR, Singh PG (2012) Intrusion detection and prevention system (IDPS) technology-network behavior analysis system (NBAS).
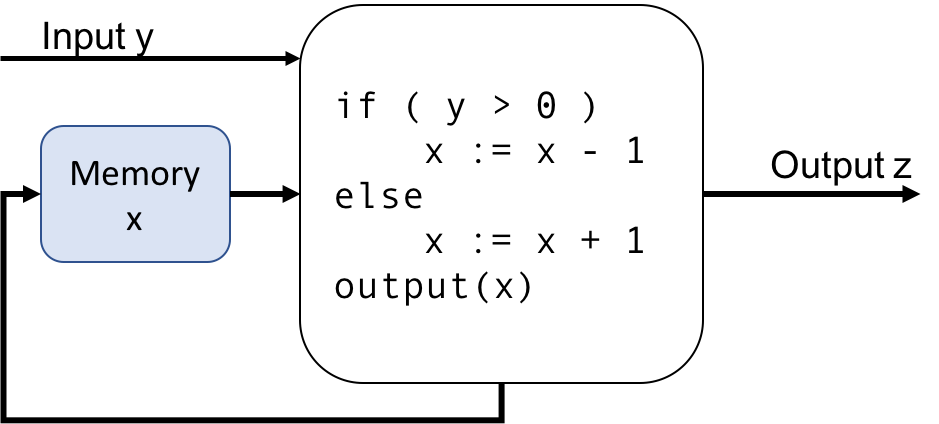
Comput Netw Int J Comput Telecommun Netw 31:2435–2463 Paxson V (1998) Bro: a system for detecting network intruders in real time. The discussions state that by effectively using these grouping algorithms along with DFA based DPI, the number of states can be reduced, providing a balance between the memory consumption, time complexity, throughput, inspection speed, convergence speed and grouping time. This study reviews and compares the various alternatives of IOGA including genetic algorithm, ant colony optimization, particle swarm optimization, bacterial foraging optimization, artificial bee colony algorithm, biogeography based optimization, cuckoo search, firefly algorithm, bat algorithm and flower pollination algorithm for solving the problem of DFA state explosion and also for improving the overall efficiency of deep packet inspection (DPI). Grouping the regular expression efficiently solves the state explosion problem by achieving large-scale best tradeoff among memory utilization and computational time. IOGAs are used to allocate the regular expression sets into various groups and to build independent deterministic finite automata (DFA) for each group. The proposed approach is called intelligent optimization grouping algorithms (IOGA), which intends to group regular expression intelligently. To overcome this problem, a new framework is proposed, and several intelligent optimization algorithms are reviewed and compared in this framework.
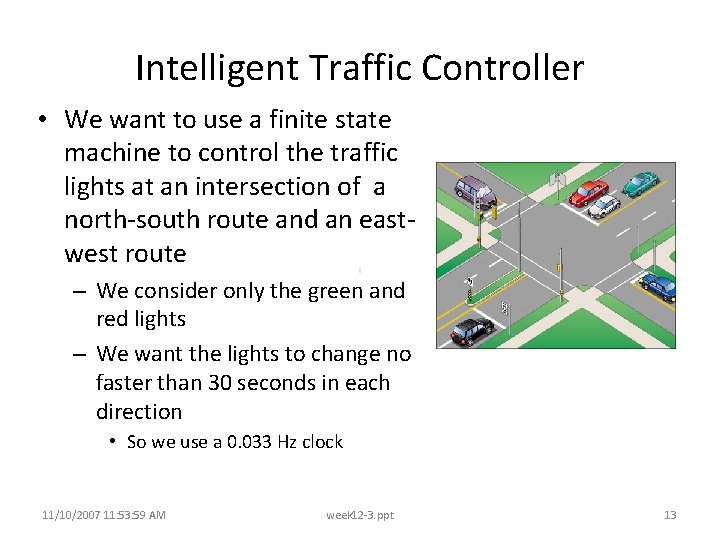
Construction and deployment of finite state automata from the regular expressions might results in huge overhead and results in the state explosion problem which is in need of large memory space, high bandwidth and additional computational time.
